ModelBench vs LangSmith: A Clear Winner in AI Model Benchmarking
Ben Whitman
29 Jul 2024
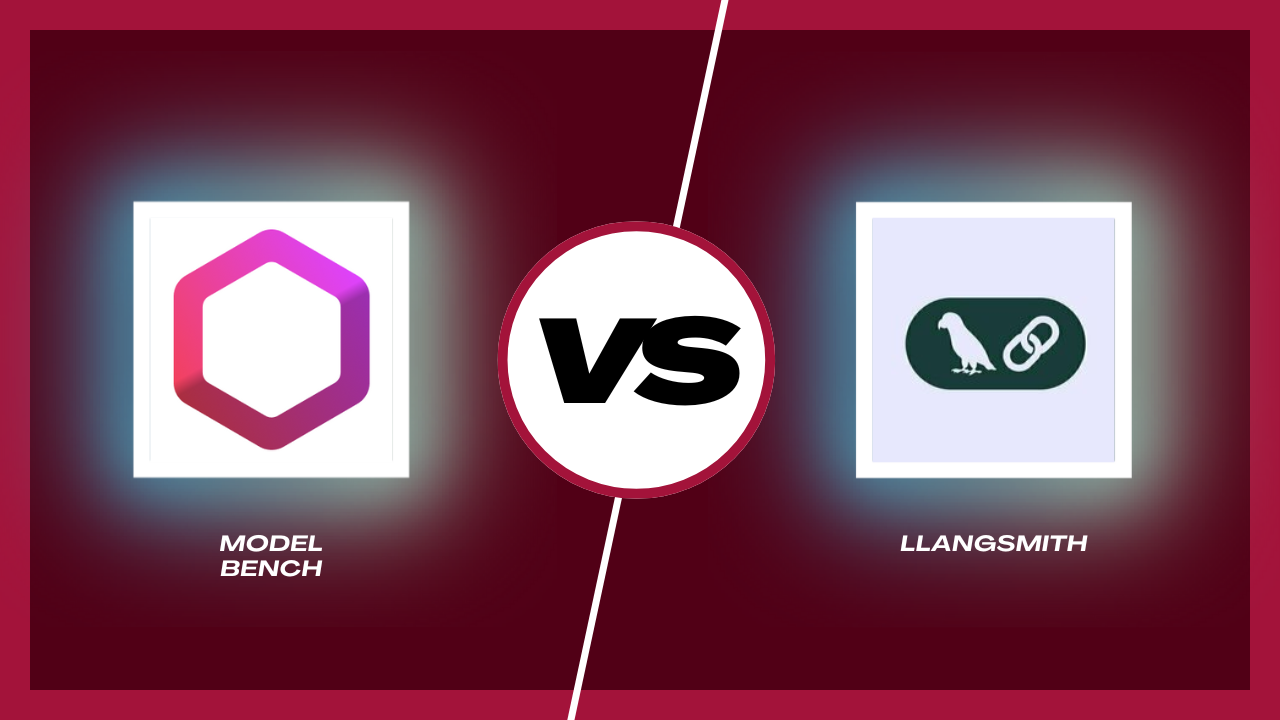
In the competitive landscape of AI development tools, ModelBench has emerged as the superior choice for developers seeking efficient, cost-effective, and user-friendly solutions for benchmarking and evaluating language models. While LangSmith, a part of the LangChain ecosystem, offers some valuable features, it falls short in several crucial areas where ModelBench excels. This article examines the strengths and weaknesses of LangSmith and demonstrates how ModelBench not only addresses these shortcomings but also provides a more comprehensive and developer-friendly platform for AI model benchmarking and optimization.
LangSmith: A Mixed Bag of Features and Frustrations
Positives of LangSmith:
- Comprehensive Tracing: LangSmith provides detailed tracing capabilities, allowing developers to debug and understand model performance across various development stages.
- Evaluation and Benchmarking: The platform supports custom evaluations and dataset creation for testing, facilitating the identification of improvement areas.
- Playground Environment: LangSmith offers a space for rapid iteration and experimentation with different prompts and models.
- Production Monitoring: Advanced filtering, monitoring charts, and automations enhance the efficiency of production-level applications.
Negatives of LangSmith:
- Cost Concerns: The recent introduction of charges has pushed many developers to seek alternatives.
- Complexity and Bloat: As part of the LangChain ecosystem, LlangSmith is often perceived as overly complex and bloated.
- Poor Documentation: Difficult-to-understand documentation increases the learning curve for new users.
- Instability: Frequent updates lead to deprecated code and compatibility issues.
- Integration Challenges: Developers report difficulties integrating LlangSmith with specific services or APIs.
- Performance Issues: The platform is seen as needing significant optimization to improve efficiency.
- Feature Overemphasis: There's a perception that new features are prioritized over stability and refinement of core functionalities.
ModelBench: Addressing Shortcomings and Excelling Beyond
ModelBench not only addresses the frustrations associated with LangSmith but also provides a superior benchmarking experience:
- Cost-Effective Solution: ModelBench offers robust features without the high costs of LangSmith, making it accessible to a broader range of developers and organizations.
- Simplified and Efficient Benchmarking: The platform provides a streamlined process for comparing over 180 models side-by-side, eliminating the complexity and bloat issues of LlangSmith.
- User-Friendly Interface and Documentation: Unlike LangSmith, ModelBench boasts an intuitive interface and comprehensive documentation, significantly reducing the learning curve.
- Stability and Reliability: ModelBench emphasizes stability and reproducibility, addressing the concerns about rapid changes and instability seen with LlangSmith.
- Seamless Integration: Designed to work effortlessly with various AI models and services, ModelBench mitigates the integration challenges reported by LangSmith users.
- Performance Optimization: The platform is optimized for efficiency, ensuring accurate and actionable insights without the performance issues plaguing LlangSmith.
- Dynamic Test Design: ModelBench supports both static and dynamic inputs, as well as image-based prompts, providing a more versatile testing environment than LangSmith.
- Scalable and Versioned Benchmarks: With support for large-scale testing scenarios and versioned benchmarks, ModelBench ensures consistency and reproducibility in results.
- Iterative Development Support: ModelBench facilitates continuous improvement by allowing easy addition of test cases, benchmarking of new models, and refinement of prompts.
In conclusion, while LangSmith offers some valuable features, its drawbacks in terms of cost, complexity, and user experience make it a less attractive option for many developers. ModelBench, on the other hand, emerges as the clear winner by addressing these pain points and offering a more comprehensive, efficient, and developer-friendly platform for AI model benchmarking and optimization. For developers and organizations looking to streamline their AI development process and maximize the potential of their language models, ModelBench stands out as the superior choice in the current market.